Build a Digit Recognizer with PyTorch
Build and train a neural network to recognize handwritten digits
- Download MNIST database of handwritten digits
- Load the data into a PyTorch tensor
- Build a neural network model
- Train the model
- Evaluate the model
- Inspect the model outputs
1. Download MNIST database of handwritten digits
import torch
from torch.utils.data import Dataset
from torchvision import datasets
from torchvision.transforms import ToTensor
import matplotlib.pyplot as plt
training_data = datasets.MNIST(
root="data",
train=True,
download=True,
transform=ToTensor()
)
test_data = datasets.MNIST(
root="data",
train=False,
download=True,
transform=ToTensor()
)
2. Load the data into a PyTorch tensor
We will only be loading subset of data for training and testing on Google Colab
subset_training_data = torch.utils.data.Subset(
training_data,
range(0, 1000) # first 1000 examples
)
subset_test_data = torch.utils.data.Subset(
test_data,
range(0, 10) # first 100 examples
)
3. Build a neural network model
!pip install jaxtyping
import torch
from torch import nn
from jaxtyping import Float
class DigitRecognizer(nn.Module):
def __init__(self):
super().__init__()
self.input_layer = nn.Linear(784, 512)
self.relu = nn.ReLU()
self.dropout = nn.Dropout(p=0.2)
self.output_layer = nn.Linear(512, 10)
self.sigmoid = nn.Sigmoid()
def forward(self, image: Float[torch.Tensor, "..."]) -> Float[torch.Tensor, "..."]:
x = self.input_layer(image)
x = self.relu(x)
x = self.dropout(x)
x = self.output_layer(x)
x = self.sigmoid(x)
return x
4. Train the model - training loop
model = DigitRecognizer()
# classification (probability based) problem, so we use cross entropy loss
loss_function = nn.CrossEntropyLoss()
optimizer = torch.optim.Adam(model.parameters())
epochs = 10
for epoch in range(epochs):
for image, label in subset_training_data:
image = image.view(image.shape[0], 784)
labels = torch.zeros(10)
labels[label] = 1
# training loop
prediction = model(image)
# cancel out previous gradients
optimizer.zero_grad()
print(prediction)
loss = loss_function(prediction, torch.tensor([label]))
# calculate every single (netowrk weights) derivative required to perform gradient descent
# derivative of loss function (error) with respect to every single parameter in the model
loss.backward()
# update the weights
optimizer.step()
5. Evaluate the model
# evaluation mode. don't worry about calculating derivative as it's not for training
model.eval()
for image, label in subset_test_data:
image = image.view(image.shape[0], 784)
prediction = model(image)
# get max from the model prediction over row (dim=1)
# max is the result
max, idx = torch.max(prediction, dim=1)
for i in range(len(image)):
plt.imshow(image[i].view(28, 28))
plt.show()
print(f'Predicted Digit: {idx[i].item()}. Actual Digit: {label}')
6. Inspect the model outputs
Predicted Digit: 7. Actual Digit: 7
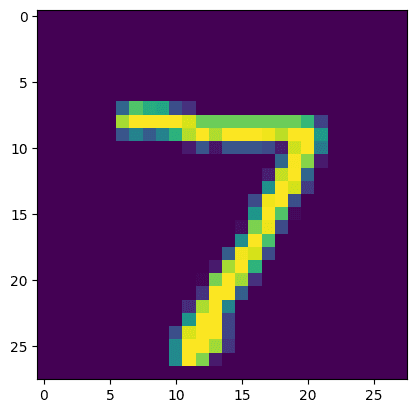
Predicted Digit: 2. Actual Digit: 2
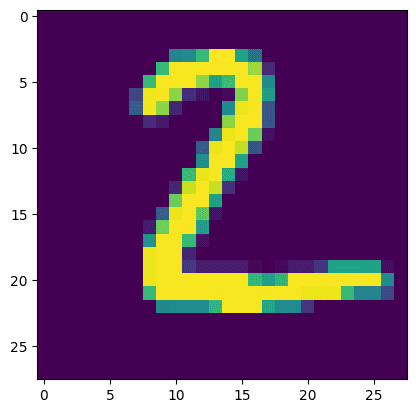
Predicted Digit: 1. Actual Digit: 1
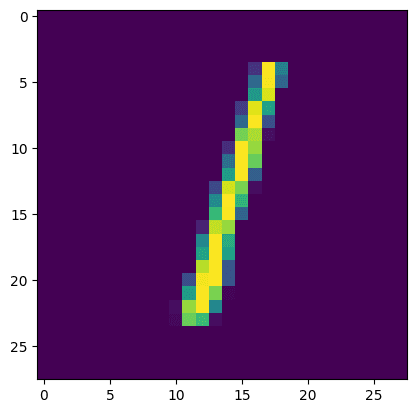
Predicted Digit: 0. Actual Digit: 0
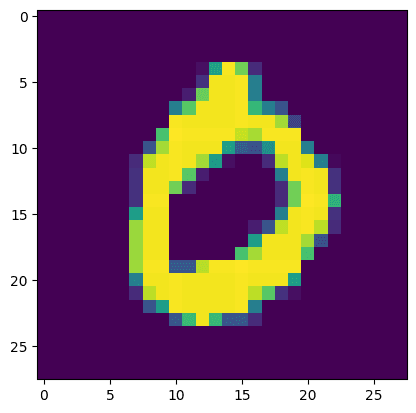
Predicted Digit: 4. Actual Digit: 4
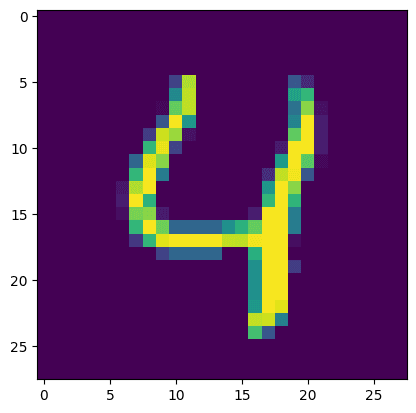
Predicted Digit: 1. Actual Digit: 1
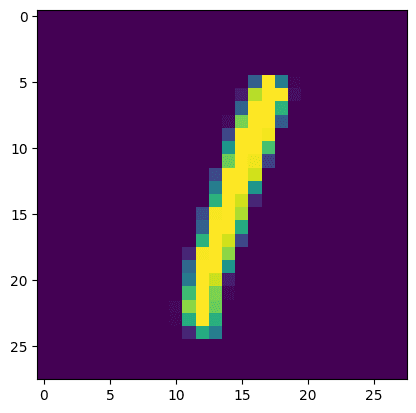
Output 1 - Predicted Digit: 7. Actual Digit: 7
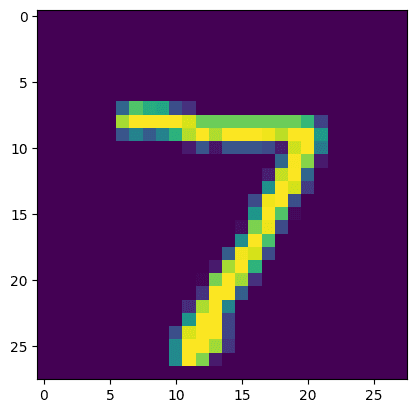